In future, we’ll see fewer generic AI chatbots like ChatGPT and more specialised ones that are tailored to our needs
- Applied Institute for Research in Economics
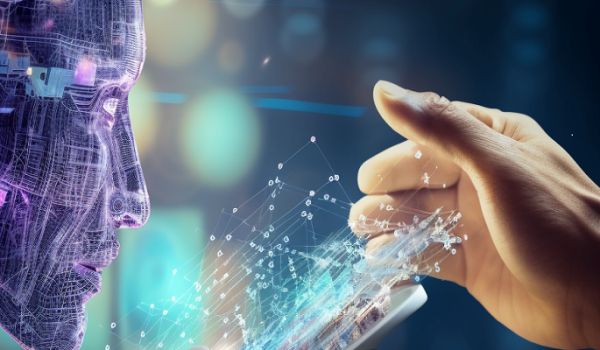
This article originally appeared on The Conversation
<p>AI technology is developing rapidly. ChatGPT has become the <a href="https://www.forbes.com/sites/cindygordon/2023/02/02/chatgpt-is-the-fastest-growing-ap-in-the-history-of-web-applications/">fastest-growing online service</a> in history. Google and Microsoft are integrating generative AI into their products. And world leaders are excitedly embracing AI as a tool for economic growth. </p>
<p>As we move beyond ChatGPT and Bard, we’re likely to see AI chatbots become less generic and more specialised. AIs are limited by the data it’s exposed to in order to make them better at what they do – in this case mimicking human speech and providing users with useful answers. </p>
<p>Training often casts the net wide, with <a href="https://www.theverge.com/2023/7/5/23784257/google-ai-bard-privacy-policy-train-web-scraping">AI systems absorbing thousands of books and web pages</a>. But a more select, focused set of training data could make AI chatbots even more useful for people working in particular industries or living in certain areas. </p>
<h2>The value of data</h2>
<p>An important factor in this evolution will be the growing costs of amassing training data for advanced large language models (LLMs), the type of AI that powers ChatGPT. Companies know data is valuable: Meta and Google make billions from selling adverts targeted with user data. But the value of data is now <a href="https://www.ft.com/content/20c27dc2-5cb6-4aa0-a6c7-71342b661a6b">changing</a>. Meta and Google sell data “insights”; they invest in analytics to transform many data points into predictions about users.</p>
<p>Data is valuable to OpenAI – the developer of ChatGPT – in a subtly different way. Imagine a tweet: “The cat sat on the mat.” This tweet is not valuable for targeted advertisers. It says little about a user or their interests. Maybe, at a push, it could suggest interest in cat food and Dr Suess.</p>
<p>But for OpenAI, which is building LLMs to produce human-like language, this tweet is valuable as an example of how human language works. A single tweet cannot teach an AI to construct sentences, but billions of tweets, blogposts, Wikipedia entries, and so on, certainly can. For instance, the advanced LLM GPT-4 was probably built using data scraped from X (formerly Twitter), Reddit, Wikipedia and beyond.</p>
<p>The AI revolution is changing the business model for data-rich organisations. Companies like Meta and Google have been <a href="https://www.ft.com/content/20c27dc2-5cb6-4aa0-a6c7-71342b661a6b">investing in AI research and development</a> for several years as they try to exploit their data resources. </p>
<p>Organisations <a href="https://www.wired.co.uk/article/twitter-data-api-prices-out-nearly-everyone">like X</a> and <a href="https://www.cnbc.com/2023/06/01/reddit-eyeing-ipo-charge-millions-in-fees-for-third-party-api-access.html">Reddit</a> have begun to charge third parties for API access, the system used to scrape data from these websites. Data scraping costs companies like X money, as they <a href="https://www.theverge.com/2023/7/1/23781198/twitter-daily-reading-limit-elon-musk-verified-paywall">must spend more on computing power</a> to fulfil data queries.</p>
<p>Moving forward, as organisations like OpenAI look to build more powerful versions of its GPT LLM, they will face greater costs for getting hold of data. One solution to this problem might be synthetic data.</p>
<h2>Going synthetic</h2>
<p>Synthetic data is <a href="https://www.ft.com/content/053ee253-820e-453a-a1d5-0f24985258de">created from scratch by AI systems</a> to train more advanced AI systems – so that they improve. They are designed to perform the same task as real training data but are generated by AI. </p>
<p>It’s a new idea, but it faces many problems. Good synthetic data needs to be <a href="https://news.mit.edu/2020/real-promise-synthetic-data-1016">different enough from the original data</a> it’s based on in order to tell the model something new, while similar enough to tell it something accurate. This can be difficult to achieve. Where synthetic data is <a href="https://sloanreview.mit.edu/article/the-real-deal-about-synthetic-data/">just convincing copies</a> of real-world data, the resulting AI models may struggle with creativity, entrenching existing biases.</p>
<p>Another problem is the <a href="https://www.axios.com/2023/08/28/ai-content-flood-model-collapse">“Hapsburg AI” problem</a>. This suggests that training AI on synthetic data will cause a decline in the effectiveness of these systems – hence the analogy using the infamous inbreeding of the Hapsburg royal family. <a href="https://www.cl.cam.ac.uk/%7Eis410/Papers/dementia_arxiv.pdf">Some studies</a> suggest this is already happening with systems like ChatGPT.</p>
<p>One reason ChatGPT is so good is because it uses <a href="https://openai.com/research/learning-from-human-preferences">reinforcement learning with human feedback</a> (RLHF), where people rate its outputs in terms of accuracy. If synthetic data generated by an AI has inaccuracies, AI models trained on this data will themselves be inaccurate. So the demand for human feedback to correct these inaccuracies is likely to increase. </p>
<p>However, while most people would be able to say whether a sentence is grammatically accurate, fewer would be able to comment on its factual accuracy – especially when the output is technical or specialised. Inaccurate outputs on specialist topics are less likely to be caught by RLHF. If synthetic data means there are more inaccuracies to catch, the quality of general-purpose LLMs may stall or decline even as these models “learn” more.</p>
<h2>Little language models</h2>
<p>These problems help explain some emerging trends in AI. Google engineers have revealed that there is little preventing third parties from <a href="https://www.semianalysis.com/p/google-we-have-no-moat-and-neither">recreating LLMs</a> like GPT-3 or Google’s LaMDA AI. Many organisations could build their own internal AI systems, using their own specialised data, for their own objectives. These will probably be more valuable for these organisations than ChatGPT in the long run.</p>
<p>Recently, the Japanese government noted that developing a <a href="https://www.taira-m.jp/ldp%E2%80%99s%20ai%20whitepaper_etrans_2304.pdf">Japan-centric version of ChatGPT</a> is potentially worthwhile to their AI strategy, as ChatGPT is not sufficiently representative of Japan. The software company <a href="https://www.sap.com/uk/products/artificial-intelligence/generative-ai.html">SAP has recently launched its AI “roadmap”</a> to offer AI development capabilities to professional organisations. This will make it easier for companies to build their own, bespoke versions of ChatGPT.</p>
<p>Consultancies such as <a href="https://www.mckinsey.com/about-us/new-at-mckinsey-blog/meet-lilli-our-generative-ai-tool">McKinsey</a> and <a href="https://kpmg.com/au/en/home/media/press-releases/2023/03/kpmg-unveils-cutting-edge-private-chatgpt-software-march-2023.html">KPMG</a> are exploring the training of AI models for “specific purposes”. Guides on how to <a href="https://bdtechtalks.com/2023/06/01/create-privategpt-local-llm/">create private, personal versions of ChatGPT</a> can be readily found online. Open source systems, such as <a href="https://gpt4all.io/index.html">GPT4All</a>, already exist.</p>
<p>As development challenges – coupled with potential regulatory hurdles – mount for generic LLMs, it is possible that the future of AI will be many specific little – rather than large – language models. Little language models might struggle if they are trained on less data than systems such as GPT-4. </p>
<p>But they might also have an advantage in terms of RLHF, as little language models are likely to be developed for specific purposes. Employees who have expert knowledge of their organisation and its objectives may provide much more valuable feedback to such AI systems, compared with generic feedback for a generic AI system. This may overcome the disadvantages of less data.<!-- Below is The Conversation's page counter tag. Please DO NOT REMOVE. --><img src="https://counter.theconversation.com/content/212578/count.gif?distributor=republish-lightbox-basic" alt="The Conversation" width="1" height="1" style="border: none !important; box-shadow: none !important; margin: 0 !important; max-height: 1px !important; max-width: 1px !important; min-height: 1px !important; min-width: 1px !important; opacity: 0 !important; outline: none !important; padding: 0 !important" referrerpolicy="no-referrer-when-downgrade" /><!-- End of code. If you don't see any code above, please get new code from the Advanced tab after you click the republish button. The page counter does not collect any personal data. More info: https://theconversation.com/republishing-guidelines --></p>
<p><span><a href="https://theconversation.com/profiles/stuart-mills-884987">Stuart Mills</a>, Assistant Professor of Economics, <em><a href="https://theconversation.com/institutions/university-of-leeds-1122">University of Leeds</a></em></span></p>
<p>This article is republished from <a href="https://theconversation.com">The Conversation</a> under a Creative Commons license. Read the <a href="https://theconversation.com/in-future-well-see-fewer-generic-ai-chatbots-like-chatgpt-and-more-specialised-ones-that-are-tailored-to-our-needs-212578">original article</a>.</p>
Contact us
If you would like to get in touch regarding any of these blog entries, or are interested in contributing to the blog, please contact:
Email: research.lubs@leeds.ac.ukPhone: +44 (0)113 343 8754
Click here to view our privacy statement
The views expressed in this article are those of the author and may not reflect the views of Leeds University Business School or the University of Leeds.