Why a holistic approach is needed to improve supply chain forecasting
- Centre for Decision Research
- Management and Organisations
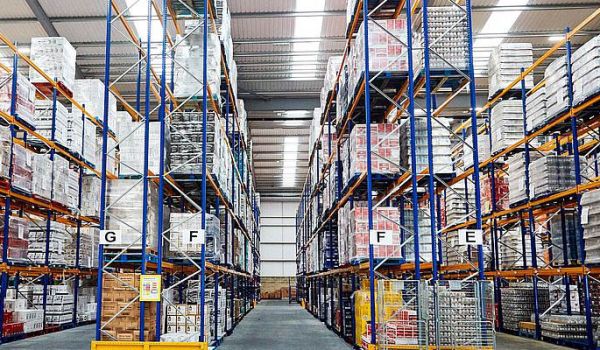
Identifying the problem areas
Over a four and a half year period, I worked for a large, international pharmaceutical company studying the organisation and working as a Business Analyst. The business was highly regulated and manufactured a low volume, high mix product that had many stages of manufacture and testing. Most of these stages had human involvement with a high level of individual responsibility.
The company used forecasts reactively on a daily basis, along with information on the inventory position, orders, and demand for calculating expected weeks on hand. This information was compared with a SKU’s expected delivery date to determine whether a given item was at risk of going into backorder. Because of this, there was a considerable sharing of information along the internal length of the supply chain.
The manufacture of the product was well honed and getting better every day due to great work within the plant to implement lean wherever possible, but the difficulties of applying lean in this complicated semi-process environment were beginning to put limits on how far they could go with improving production planning, quality control and testing.
We know that a small improvement in forecast, if it is effectively fed through into production and the rest of the supply chain, can have a massive effect on inventory holdings and production variability, saving money and increasing efficiency. Often overlooked is that these improvements can also help the stakeholders to feel more in control of their work and less stressed on a daily basis so that a virtuous cycle is established.
Within the company, the highly regulated environment tended to foster a silo mentality and the demands of daily work with such a complicated product meant that people had little time for cross-functional discussions. Because of this, misunderstandings occurred, for instance, Quality Control thought that the frequent requests for them to push items through was due to bad forecasting, Testing thought that their capacity problems were due to poor scheduling, and Scheduling thought that if an item was difficult for one area it would be the same for everyone in the post production testing and quality control process.
There had been work to use Statistical Process Controls in some areas but, for some, this just felt like more pressure and that essential information was not being considered, like capacity limitations and levels of difficulty.
Implementing key learnings
From working within the company, analysing their data (which included sales, lead times, shelf lives and associated master data), observing behaviours and holding workshops with employees, I identified ways the organisation could improve the accuracy and efficiency of their supply chain.
Firstly, I performed a forecast audit while working closely with the demand planners and their managers every day. This improved understanding of effects like intermittency in the sales data and aggregation.
We explored the Bullwhip Effect which prompted behaviour change and changes to practice. We also discovered new metrics which helped everyone in the factory to focus on the customer and these came about through using higher velocity, finer grained data with good master data development. I say ‘we’ because whilst I contributed the academic knowledge and the data analytics tools, the stakeholders shared their day to day difficulties with me, and told me what they would love to be able to do, together we got as close to a perfect end as we could.
These improvements were specific to demand planning but I, and the senior management I was working with, wanted to know how viable a process of level loading the factory would be and for this I chose to use a technique known as PartiSim © (a participative simulation framework). The technique provided ways to get cross-functional groups together to explore how they felt about the idea of production smoothing and what they experienced in their day-to-day working processes that stopped them from being smooth. It helped us to explore the veracity of their data, since data does not always say what one expects and never tells us the whole story.
I made analytical visual models based on what the entire cross functional group was telling me, which allowed us to agree on a conceptual model and what data we would use in it. We built a simulation model that showed how long items were taking as they moved through the factory which led to a simplified version being used by the senior management to make major decisions regarding production and planning management.
Seeing improved results
After embracing the socio-technical aspects of forecasting systems and making the necessary changes to their supply chain, the company I worked with saw a number of positive results.
Further improvements came about due to the model developments cross functional and open nature (e.g. the expression of feelings and experience in the company of other functions) using data to explore processes. For instance, the Schedulers learned that the level of difficulty was not the same for everyone, the Quality Controllers found out that customer behaviour made demand difficult to manage and that the forecasting was pretty good all things considered, and they all realised that they were all trying to reschedule based on a fear of going into backorder which was causing disruption and not making backorder less likely.
They realised that they did not need to be this reactive, that they could simplify their processes and that it could be made more stable. They also learned about ways to help each other out and started doing so, one person in planning even started creating new analytics tools for others to use in testing and quality control. Most of all, the culture changed to become more open, inquisitive and data driven.
After implementing these changes, the company achieved large savings on inventory and scrap; it was estimated that the improvements had contributed to inventory savings of €4m and a 40% increase in inventory turns. The head count also reduced by approximately 30% in planning, as workloads eased due to the smoothing effects induced by the work.
It’s more than just data and tools
These changes could be applied to other semi-process industries that are having difficulty in applying lean due to the variability in their processes. Lean is not just tools but it is concepts as well, such as variability reduction. When using techniques like this we can enable continuous improvement such that staff can understand the limits of their process variation and creatively design mutually beneficial ways of managing it which use data driven methods.
By not just relying on the data but also encouraging staff to understand how their role in the supply chain affects the whole process, manufacturers can improve the accuracy and quality of forecasting. The very process of working with the social aspects of the system as well as the data can have unexpected positive results as it gives an opportunity for cross-functional alignment to occur and prompts change from within.
Contact us
If you would like to get in touch regarding any of these blog entries, or are interested in contributing to the blog, please contact:
Email: research.lubs@leeds.ac.uk Phone: +44 (0)113 343 8754
The views expressed in this article are those of the author and may not reflect the views of Leeds University Business School or the University of Leeds.