What Can Elite Sport Teach Us About Effective Data Analytics?
- Management and Organisations
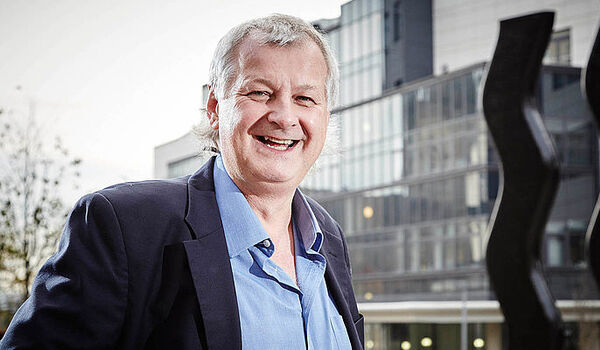
Elite sport is at the leading edge in the development of data analytics. A CEO of a leading analytics consultancy recently claimed that “the world of sport is 20 years ahead of the business world in terms of how to get the best out of your performance by analysing data”. Hollywood has even made an Oscar-nominated movie on sports analytics. Moneyball, starring Brad Pitt, tells the story of how the Oakland A’s, the Leicester City of Major League Baseball, use data analytics to identify talented but affordable players overlooked by richer teams.
I have worked on applying data analytics in elite sport for over 20 years. My work has encompassed everything from player valuation to performance analysis. I have worked with teams around the world in a variety of sports including football, rugby union, rugby league and Olympic cycling. There are eight lessons that I would take from my experiences in elite sport on how to use data analytics effectively to improve performance in any organisation.
Lesson 1: Analytics must always be decision-driven not data-led or technique-led.
Analytics is actionable insight. It is data analysis undertaken for the sole purpose of supporting management decision making. All analytics projects must start with a clear understanding of the management decisions that will be informed by the analysis. Decisions must drive data collection and choice of analytical techniques, and not vice versa. Some data analysts often seem more concerned with using the most complex analytical technique, the biggest dataset and the newest software. This is doomed to disaster, producing sophisticated analysis with zero impact on the end-user. Over the years I have met several frustrated analysts complaining that they had produced great analysis but it had been ignored by the coaches. Invariably the coaches had no input in the initial planning of the analysis so their lack of engagement with the output is hardly surprising.
Lesson 2: Analytics can only be effective in organisations with an evidence-based culture.
Analytics can only be truly effective in organisations with a strong commitment to an evidence-based approach. Thomas Davenport, one of the gurus on the management implications of data analytics, contrasts analytically-minded organisations with those that are “knowledge-allergic” in which managers rely on their gut instincts, preferring anecdote and assertion to analysis. Organisations using analytics effectively have got buy-in to the evidence-based approach at all levels and in all departments. At Saracens, now one of the leading teams in European rugby, the evidence-based culture was instigated by the previous Director of Rugby, Brendan Venter, a former South African World-Cup winner and a qualified medical practitioner who adopts the same evidence-based discipline to coaching rugby as he does to treating his patients. Be it the search for better health, or better playing performance, Venter believes wholeheartedly in interrogating the available evidence before deciding on the appropriate course of action.
Lesson 3: Analytics should result in data reduction rather than adding to data overload.
The problem facing all organisations is that the technology to collect data has developed faster than the capacity to use the available data in a meaningful way. This puts a real premium on data analysts who are able to identify the really important data. Analysts must filter out the irrelevant data rather than just collecting and producing more data that just exacerbates the data overload problem. Currently I am assisting a European football club to develop their use of data analytics. After every round of games in their domestic league I receive spreadsheets for each game with around 7,500 rows of detailed data covering every action in the game. I also receive around 200 metrics on every player who has played. My task is reduce all of this data to a game report with summary tables, accompanying graphics and a single page of key points.
Lesson 4: Ultimately data analysis is a signal-extraction process.
Analysts are skilled at decomposing a mass of data into systematic variation (the informative “signal”) and random variation (the meaningless “noise”). It is a signal-extraction process with analysts continually checking that their models are fit for purpose, capturing as much of the informative signal as possible and ensuring that none of the signal is wrongly classified as meaningless noise. Getting it wrong can be very costly and undermine rather than improve performance levels. Elite teams have very limited preparation time for games during the season so it is vital to use training to work on areas of systematic weakness and to avoid over-prioritising an area on the basis of a single random blip in performance.
Lesson 5: The most important data are the expert data created within an organisation.
Improving performance service requires focusing on the controllables – are people in the organisation doing the right things, and are they doing things right? Effective managers facilitate improved performance by helping people to develop their skills and to make better decisions in a given situation. So the most important data for an organisation is the expert data that only its own managers can produce on the quality of the decision making and skills of the people they are responsible for managing. The most valuable data that football and rugby coaches produce are their own expert evaluations of player technique and the extent to which players implement the game plan by making the right decisions in game situations.
Lesson 6: Analytics is not all about big data.
So much of the current focus in analytics is on discovering meaningful relationships in really big datasets. But we should never forget the value of small data. Analytics is about supporting decision making by using the available evidence as effectively as possible, irrespective of the size of the dataset. Some of the most valuable datasets are often small in their width of coverage but contain great depth of detail. Statisticians teach us that datasets with under 30 observations are too small to produce reliable results but if I waited until a team played 30 games before producing any analysis it would be near the end of the season and far too late for any analysis to have an impact. Analytics is about the practicalities of applying statistics in a real-world setting, making the best use of the data we actually have.
Lesson 7: Analytics is mostly exploratory and explanatory, seldom predictive.
I am often asked if sports analytics could produce an algorithm for predicting games with obvious possibilities for betting on games. Apart from the regulatory and ethical issues, it is highly unlikely to be a winning strategy since betting companies employ data analysts to build algorithms for setting the optimal odds on game outcomes. When it comes to gambling, remember the house always wins in the long run because it has better information and better analysis than most of the punters. More importantly, linking analytics and prediction often betrays a belief that analytics is about predicting the future. It isn’t. Analytics explores data to help provide a better understanding of what has happened and why so as to help guide decisions on future actions. Analytics is predictive only to the extent that it extrapolates current behaviour patterns into the future. If behaviour patterns change, extrapolations are rendered worthless. Analytics can only detect behavioural changes after the event. So beware of analysts claiming to provide predictions about how the world will change. They are selling you snake-oil.
Lesson 8: Effective analysts are humble servants who respect the experience and expertise of the end-users.
Analytics exists to support decision makers and, to be effective, analysts must continually engage with the end-users. Not only should analysts be very clear on which decisions they are expected to inform, they should also tap into the detailed knowledge that the end-users have of the specific context in which they are operating. Analysts must avoid being seen as the “Masters of the Universe” who think that they know it all because of their sophisticated mathematical and statistical abilities. As the old Chinese proverb goes, “knowledge is knowing what you do not know”. Respect the end-user, work for them and with them, and always be very clear on what you as an analyst can bring to the table (the ability to forensically explore data) and what you don’t (experience and expertise of the specific decision context). Effective analytics is a team effort.
Bill will be presenting on the effective use of data analytics in rugby union at the Rugby Innovation Summit to be held at the Twickenham Stoop Stadium, London on 24 and 25 May 2016.
Contact us
If you would like to get in touch regarding any of these blog entries, or are interested in contributing to the blog, please contact:
Email: research.lubs@leeds.ac.uk Phone: +44 (0)113 343 8754
The views expressed in this article are those of the author and may not reflect the views of Leeds University Business School or the University of Leeds.